Core Protocol Vision
Motivations + principles
In our modern high-tech society, computing is ubiquitous, though surprisingly cumbersome to utilize for the everyday person beyond just pre-defined use-cases. Personally, I bought a few Raspberry Pis and other mini computers with creative intentions to automate some reasonably useful but not essential things at home. Like controlled heating for my wife’s hummingbird feeder, to keep it from freezing in the winter. But in the end, multiple projects didn’t make it, because keeping the Raspberry up to date and running requires some regular time for maintenance.
What is missing is a secure computing and data-storage platform where various simple software applications can easily interact and I can add customized software extensions or new components, without me having to set up and maintain the platform my programs run on.
Sharing your own photos or other data with your neighbor is easily possible via the internet, without needing to install a networking cable. Running your customized computation is intractable for most, except hobbyist enthusiasts and professionals, because it requires difficult setup of dedicated hardware. In the future, where devices are getting more intelligent and adaptable to their owners' individual living patterns, we’ll need some computing platform where individuals can easily compose their coordination and control applications and interface with other’s. Now imagine there is an extension of the internet adding moderate compute and data storage capabilities.
Global distribution is necessary as the internet’s utility as a global communication network is primarily determined by its reach. Decentralized operation by a geographically diverse group of organizations is foundational for the internet’s impartial nature to special interests, and therefore its global adoption as universally-used infrastructure.
Since the internet already provides the means to exchange data, attached computation and data-storage services can be geographically or operationally centralized, as is the case with cloud providers. Nevertheless, over the last years we have learned the values of diversity and resilience: geographic diversity generates resilience against natural disasters, wars, pandemics, and autocratic governments; operational diversity strengthens resilience against technical vulnerabilities or bugs as well as narrow corporate or political interests. For example, due to centralization concerns about the StarLink satellite network, the European Union just decided to develop its own version IRIS² [0]. Therefore, we believe that decentralized operation by a globally-distributed group of organizations is crucial for Flow’s resilience and its impartial nature. Especially the latter is critical for large-scale adoption without fearing that access restrictions could be leveraged for economic or political power struggles.
In technical terms, a system is called ‘Byzantine Fault Tolerant’ [BFT] [1, 2] if it continues to work correctly despite some of its components actively maliciously trying to compromise it. In the case of the internet, you don’t have to trust the operator of the deep-sea cable when you send your credit card details across the Atlantic for an online purchase. Data integrity is guaranteed by common internet protocols (provided your browser follows best practices), even if the deep-sea cable operator is compromised and aiming to tamper with your data. Therefore, I would classify the internet as a BFT data transmission network. However, attached computing and data storage services are generally not BFT, limiting their resilience.
Similarly, being BFT is critical for Flow by allowing collaborative, globally-distributed operations by diverse operations without creating single points of failure.
Being an efficiently-scalable platform for general-purpose computation is the last set of requirements. You have a user account (or multiple) on Flow, where you can install programs and store data – very similarly to a user account Linux, Windows, or MacOS. Write access to data is strictly controlled, so that by default only the owner of the account can modify the data and other accounts only with explicit permissions.
Key concepts and terminology for a globally-distributed, fault-tolerant, scalable operating system
Nodes
Nodes are the building blocks that collectively form our globally-distributed, BFT, open operating system. Informally, a node can be described as an individual actor. It may be a single server or a group of many microservices depending on the node’s responsibilities. The important aspect is that a node is a single entity that either plays by the rules or is considered malicious (formally ‘byzantine’). Nodes are run by ‘node operators’, typically companies, non-profit organizations, governments, or individuals with sufficient domain expertise.
Protocol
The protocol specifies how nodes are supposed to interact. An ‘honest’ node always follows the rules, which includes being available to accept work.
Byzantine node
A malicious or byzantine node can deviate from the protocol in any way it desires or be offline [1]. A byzantine node may behave honestly for a very long time and only reveal its malicious intent at some point later (or never) potentially in a coordinated attack together with other byzantine nodes. A system is Byzantine Fault Tolerant, if it continues to produce correct outputs even if byzantine nodes are participating.
Motivations for Flow
Flow should scale to global adoption by 1 billion users and be capable of running frequently-interacting, potentially safety-critical applications with moderate computational and data storage requirements. Algorithmically, Flow belongs to the family of blockchain protocols. Though, we feel there is much value in putting a strong focus on our final goal: engineering a globally-distributed, efficiently scalable, BFT operating system. Throughout Flow’s design and architecture, we consequently applied the following guiding principles that are all motivated by this end goal.
Usability: absorbing complexity into the platform instead of outsourcing it developers
From our perspective, the following best practices broadly strengthen usability, which Flow implements today except for the last item, which is on our roadmap.
- We believe that consistency brings a huge benefit for a software developer. Working with eventually-consistent databases or implementing concurrent algorithms substantially increases software complexity, engineering time and maintenance cost [3]. In technical terms, we desire ACID-compliant transactions.
- An intuitive concept of a user account is very important, where an account can have multiple access keys with potentially different permissions, keys can be cycled, etc.
- We desire a programming language, where objects have a native notion of access permissions. Thereby, we can represent concepts like “You give me an object, where you as the creator determine what I can do with the object (including whether or not I can copy it).” Cadence is Flow’s programming language with native language features to express ownership and access permissions.
- Lastly, having a (cryptographically secure) pseudo random number generator [PRNG] natively within the system is immensely useful. Therefore, Flow implements a DFinity-style random beacon [4] (including a distributed key generation). Among other low-level protocol functions, the Flow Virtual Machine utilizes the random beacon to seed PRNGs for smart contracts. The resulting random numbers are unpredictable, verifiable and tamper-proof – all in the presence of a colluding group of adversarial nodes within the network [5].
By implementing broadly-used functionality on a platform level, such random number generation, we reduce development overhead. Developers can focus on their core goals, instead having to work around shortcomings of the platform.
Efficiency as a fundamental requirement
In 2020, researchers estimated that information and communications technology causes approximately 2% - 4% of global greenhouse gas emissions [6]. In addition, there is a substantial ecological impact from extracting and refining the required resources, such as cobalt, copper, lithium and rare-earth elements. Therefore, efficient resource consumption is a central architectural metric for Flow.
In 2021 Flow consumed a total of 0.18 GWh in energy [7], which equals the power consumption of about 30 people [8]. Already today, Flow is among the most efficient BFT compute platforms in existence [9]. As Flow matures and transaction load increases, we expect Flow’s scaling efficiency to generate a more pronounced advantage.
We believe that mass adoption requires significant scalability, in particular with respect to throughput, computational resources and user data. There are roughly 5 billion internet users today. A billion user accounts on average storing 1MB of data each, would already amass to 1 petabyte of data for a single snapshot. Maintaining a limited history of the most recent changes, which is common in highly-distributed fault-tolerant systems for the sake of resilience, further exacerbates the storage requirements. Moreover, we want to support that accounts store much more than 1 MB of data if warranted by the use case.
Furthermore, we believe that a global operating system would need to process up to 1 million transactions per second. In Flow, a transaction is essentially a little general-purpose program. It can do as little as just calling some function of an application already running on the platform. But a transaction can also contain a more complicated program that interacts with a variety of other applications on Flow. In academic literature it is common practice to work with an average transaction size of 512B [10], which we believe is already a conservative estimate for a general-purpose compute platform. Already in this scenario, Flow would need to ingest 0.5GB of data per second.
At the moment, we utilize composability heavily at the compile stage, where the software is assembled from various independent libraries. Microservice systems are another example, but here the composition happens at runtime. Composability, reusability and collaborative improvement and evolution are the central benefits of open source software development.
There is a strong trend towards software as a service, where the client interacts with software instances that the software developer operates. Unfortunately, the currently prevalent deployment of software as a service is on closed infrastructure. This creates substantial barriers for software composability, because every aspect of customizability by the user must be coordinated with the software provider. You can’t easily bring your own plugins or extensions to software running as a service, as this would not only require to implement the designated interfaces (the easy part) but generally also compatibility with the proprietary, closed infrastructure of the software provider.
Highly composable and customizable software has been identified as a key property for future enterprise applications [11, 12, 13, 14]. Also as individuals, we evolve towards composing software applications according to our individual preferences and needs; for example your personal mix of interacting apps on your smartphone. Open software execution extends the benefits of open source to the runtime environment. You can bring your own plugins and software extensions to customize running software according to your needs without having to worry about deployment. The underlying idea of open execution is for software to interface dynamically at runtime depending on the needs of the individual users. Conceptually, this is the idea behind dynamically linked libraries in Windows or shared objects in Linux.
Supporting broad composability implies on a technical level that Flow has to efficiently run a network of frequently-interacting, turing-complete applications. As we illustrated in the figure below, heavily utilizing composability entails a significant amount of communication if the applications are horizontally scaled across several servers.

The scalability requirements we have outlined in the previous paragraph are quite substantial. While realizations for specialized tasks exist, such as distributed databases, there is no general-purpose BFT computation platform that can shoulder such work loads today. Limited scalability fundamentally limits usability because it simply renders a large number of interesting use cases impractical.
Furthermore, overhead in resource consumption caused by inefficiencies also scales with the system size. Needing 20% more hardware and energy for a small computational platform is often acceptable, while wasting 20% of resources in a globally-used infrastructure is not viable. Even worse, overhead often increases disproportionately when scaling up a system, e.g. 20% inefficiency in a small system increases to 30% losses in a scaled-up system.
In summary, we strongly believe that efficient scaling to very large computational loads is a fundamental prerequisite. Most aspirational software creators and companies are aiming to reach millions to billions of people. Therefore resource-efficient scalability should be an important criterion from future-proofing point-of-view when selecting a computational platform to build on.
Pipelining as Key to Scalability
We discussed the benefits and potential large-scale applications of a globally-distributed, byzantine fault-tolerant operating system from the perspective of IT security and infrastructure resilience. We introduced Flow as an archetype of such an operating system and derived the following ground-breaking scalability goals:
- Throughput of at least 1 Million transactions per second (TPS). We anticipate that this transaction volume will require the platform to ingest at least ½ gigabyte of data per second; more realistically 1 GB and upwards.
- Very large state of one Petabyte (size of state snapshot at a single block) and beyond;
- Support for large-scale software composability, i.e. running frequently-interacting, turing-complete applications
Now, we analyze the tradeoff between redundancy, resilience and scalability. We will conclude that the cost and benefits of high redundancy vary drastically throughout the lifecycle of transactions. Thus, Flow implements a modular pipeline for processing the transactions, which allows to tune the level of redundancy for each step independently.
We emphasize that scalability is one of the most fundamental and persistent challenges for distributed, BFT operating systems, including Flow and all other smart-contract-focused blockchain platforms. Therefore, we strongly believe that high-scalability requirements must be de-risked very early in the development process, being considered at every step in the architecture. Otherwise, one would outsource the risks of limited platform scalability to developers and users.
A defining feature for a byzantine-fault-tolerant compute platform is its ability to produce correct results despite a noteworthy fraction of nodes failing or being compromised. To quantify “how resilient” a BFT system is, we can look at the number of nodes that can be compromised without jeopardizing the system's overall correctness guarantee. In the blockchain domain, this number is also called Nakamoto Coefficient [16]. Conceptually, we desire this number to be large. Thereby the infrastructure becomes resilient to natural disasters, large-scale malware infections, or acts of cyberwarfare. Note that delivering correct results is a fundamental requirement, while we accept some performance degradation in case of natural disasters or large cyber attacks.
In computer-scientific literature, algorithmic guarantees are frequently of the form: “The algorithm continues to produce correct results as long as less than x% of nodes are byzantine.” This illustrates a fundamental connection between redundancy and resilience. The more redundancy there is within a system, the more nodes (absolute number) have to be compromised for the system to break.
We always need some level of redundancy, which essentially means having multiple nodes each process the same work, because any node individually might fail. Unfortunately, resource consumption (hardware and energy) increases linearly with redundancy. Therefore, for efficient scalability, it is critically important to pay close attention to where redundancy is really indispensable for resilience.

Transaction order fundamentally influences the computation outcome. Imagine there is one concert ticket left and Alice and Bob are both attempting to buy it. The person whose transaction is processed first gets the ticket, and the other’s purchase attempt fails. Clearly the order is important here. Though, it should be noted that there is no proper notion of a “correct order”. A server closer to Bob might receive his transaction first, while another server in Alice’s town could first see her transaction. In other words, both orders [transactionAlice, transactionBob] as well as [transactionBob, transactionAlice] are legitimate and Flow needs to pick one. Formally, an algorithm that solves this problem of agreeing on an order belongs to the family of consensus algorithms. The process of determining a transaction order is called ‘transaction linearization’.
It is intuitive that a single potentially malicious actor controlling the transaction ordering can exert undue influence, e.g. systematically disadvantaging some users’ transactions or censoring them entirely. Furthermore, it is very difficult to impossible for other honest nodes to conclusively prove that the transaction linearization process has been compromised, because there is no individual correct transaction order. Therefore, for the sake of resilience, we desire a larger number of nodes to be participating in consensus, i.e. a large factor of redundancy.
From the perspective of resource consumption, consensus requires only light computation. Communication latency and data exchange volumes are the main bottlenecks, especially if you have many nodes participating, which we desire for resilience. Therefore, the cost of redundancy is actually very moderate for the consensus stage, if we can keep data volumes smaller that individual consensus participants ingest (we’ll soon explain how to achieve this).
In comparison, transaction execution is very resource intensive. As discussed above, we anticipate that Flow’s system state at maturity is easily on the order of a petabyte. Furthermore, there is at least half a gigabyte of code to be executed and data to be processed per second, likely more. To shoulder such computational load, very very powerful hardware is needed, with a substantial resource footprint and massive energy consumption.
Having transactions redundantly executed by multiple hundred or even thousands of nodes is economically nonsensical and ecologically prohibitive for a computational platform of global scale. Moreover, the benefits of high redundancy are limited for the following reason. Unlike consensus, where many different correct outputs exist for the same input set, transaction execution is fully deterministic. In other words, for a given starting state and a sequence of computational instructions, there is a single objectively-correct output. If the Flow protocol has a way to assert correctness of transaction execution before it accepts the result, it becomes intractable for a malicious actor to compromise the system’s correctness by publishing wrong execution results. Therefore, correctness of the system outputs is guaranteed despite low redundancy.

Separating transaction linearization from execution is an established approach in distributed databases. We published a paper in 2019 extending this approach to computational platforms [17] and implemented it from the start in Flow. Similar ideas are being explored since 2021 by Ethereum in the context of proposer-builder separation [18] and as of 2022 also by Oasis and Infura [19]. Though, neither project has reached a finalized design yet.
Scaling Transaction Linearization via Hierarchical Consensus
In the previous section, we have seen that pipelining is key for platform efficiency. This chapter focuses on the first pipeline step: transaction linearization. We explain how Flow’s consensus system scales beyond the networking capacity of individual servers, which is unmatched in the blockchain space to the best of our knowledge.
Consistently ingesting at least half a gigabyte of transaction data per second on a single node is quite challenging and requires very powerful hardware. We don’t want to rely on a “few strong nodes” for transaction linearization, because this would compromise BFT and decentralization as explained in the last section. Conceptually, the answer to this dilemma is intuitive: individual nodes only ingest a subset of all incoming transactions.

Flow realizes horizontally-scaling transaction ingestion through a hierarchical process illustrated in Figure 3. We briefly summarize the process here and refer interested readers to our paper [20] for details. In a nutshell, there is one class of nodes called ‘collectors’, whose job is to create batches of linearized transactions. The large set of collectors (potentially a few thousand in the mature, maximally scaled protocol) are partitioned into smaller groups (50 - 100 nodes), called clusters, on a weekly basis. Each transaction goes to one particular cluster depending on its hash. If we have k clusters in the protocol, each cluster only needs to ingest 1/k of the global transaction data. A single batch, called collection, of linearized transactions might contain as many as 1000 transactions. The full collection goes directly to the Execution Nodes once enough collectors from the respective cluster have signed it and thereby promised to store the collection. However, only a reference to the collection, called collection guarantee, is sent to the consensus committee. The consensus nodes linearize the collection guarantees and put them into blocks. As an individual collection guarantee is only about 128 bytes, consensus participants can ingest thousands of them per second with light hardware requirements. The resulting processing pipeline takes the form depicted in Figure 4.

Early 2020, we published a paper describing the approach of hierarchical, horizontally scalable transaction linearization [20], as we were finalizing the corresponding collector implementation in Flow. Since mid 2021, Ethereum is thinking in a conceptually very similar direction, though without tangible release dates, using the keywords ‘data availability shards’ [21, 22] or more recently ‘danksharding’ [23, 24].
Parallel execution for horizontal scaling
After describing how to scale linearization to 1 Million transactions per second (previous chapter), we now shift our focus to transaction execution. This chapter explains how Flow achieves massive efficiency and scalability benefits by concentrating execution within a limited number of dedicated strong nodes.
We believe that an operating system tailored towards high software composability provides tremendous benefits to developers and catalyzes creativity, which in turn benefits users and fosters platform adoption. Developers heavily utilizing composability require the computing platform to efficiently run a network of frequently-interacting, turing-complete applications. With computational load approaching the anticipated levels (petabyte state and gigabyte(s) per second inbound transactions), no single server would be capable of storing the state entirely or computing all transactions at acceptable speed. Hence, transaction execution needs to scale horizontally, i.e. applications must be distributed across servers under the hood.

Applications heavily utilizing composability (figure 5a) result in significant communication volume. In this scenario, data exchange between the servers needs to be highly efficient. Otherwise, the computational platform cannot be scaled without resource consumption becoming impractical.
In comparison, rarely interacting applications (figure 5b) can be distributed across multiple servers even if data exchange between servers is resource intensive. Limiting a computational platform to sparsely-interacting applications is a convenient shortcut for the platform developer. However, thereby they outsource significant complexity to developers, because many developers have to now work around shortcomings of the platform. For a platform supporting only sparsely-interacting applications, scalability challenges have to be addressed over and over by many developer teams separately, instead of solving them once on the platform level.
The Flow team is a strong believer of absorbing complexity into the platform instead of outsourcing it to developers. We architected Flow to support large-scale composability and describe in the following how Flow efficiently scales transaction execution horizontally.
Flow employs a very limited number of dedicated execution nodes (8 to 10 max in the mature protocol). We envision that each execution node would be a small data center. Execution nodes are fully redundant to each other, meaning every execution node holds the entire state locally and executes all transactions. Our approach has the following ground-breaking efficiency benefits (also illustrated in Figure 6):
- Due to high data locality, i.e. short communication distances, limited hardware and energy resources are needed for data exchange at scale. Moreover, within the data center, specialized network infrastructure can be used that would be cost-prohibitive over longer distances, thereby further minimizing resource consumption.
- Spatially dense computation implies low-latency communication. Thereby, Flow can run heavily-interacting applications. The same computation would bottleneck on latency if executed on a widely-distributed network where servers communicate over the internet.
- As an execution node is operated by a single operator, the servers within the execution node trust each other. Thereby, the servers can retrieve state and exchange application data without relying on CPU- and energy-intensive signatures and cryptographic correctness proofs.
- The Flow protocol is oblivious to the specifics of how execution nodes internally distribute the workload across their numerous servers. The only requirement is that the execution result must be identical to sequential execution of the linearized transactions. Hence, execution nodes may internally re-order transactions and execute them in parallel, as long as the result remains invariant. This is a game-changing advantage of Flow over most other protocols for the following reasons:
• Different execution nodes can employ different heuristics for parallelizing the transactions. Most likely, there is no single algorithm for parallelizing transactions that is optimal in all scenarios. With a diverse mix of heuristics, different execution nodes will be temporarily faster than others depending on the currently prevalent load patterns. The speed of the overall platform is determined by the fastest execution node(s). Hence, diverse execution heuristics make the Flow protocol faster.
• Execution nodes can locally evolve their heuristic without needing to coordinate time-intensive protocol upgrades. Execution nodes are rewarded for speed (a topic, which we will cover in a future article) and they can treat their heuristic for parallelizing execution as intellectual property. Therefore, we expect continuous, fast-paced optimizations of speed and efficiency.
• Horizontal scaling of transaction execution being an implementation detail of the execution node also dramatically simplifies the Flow protocol.
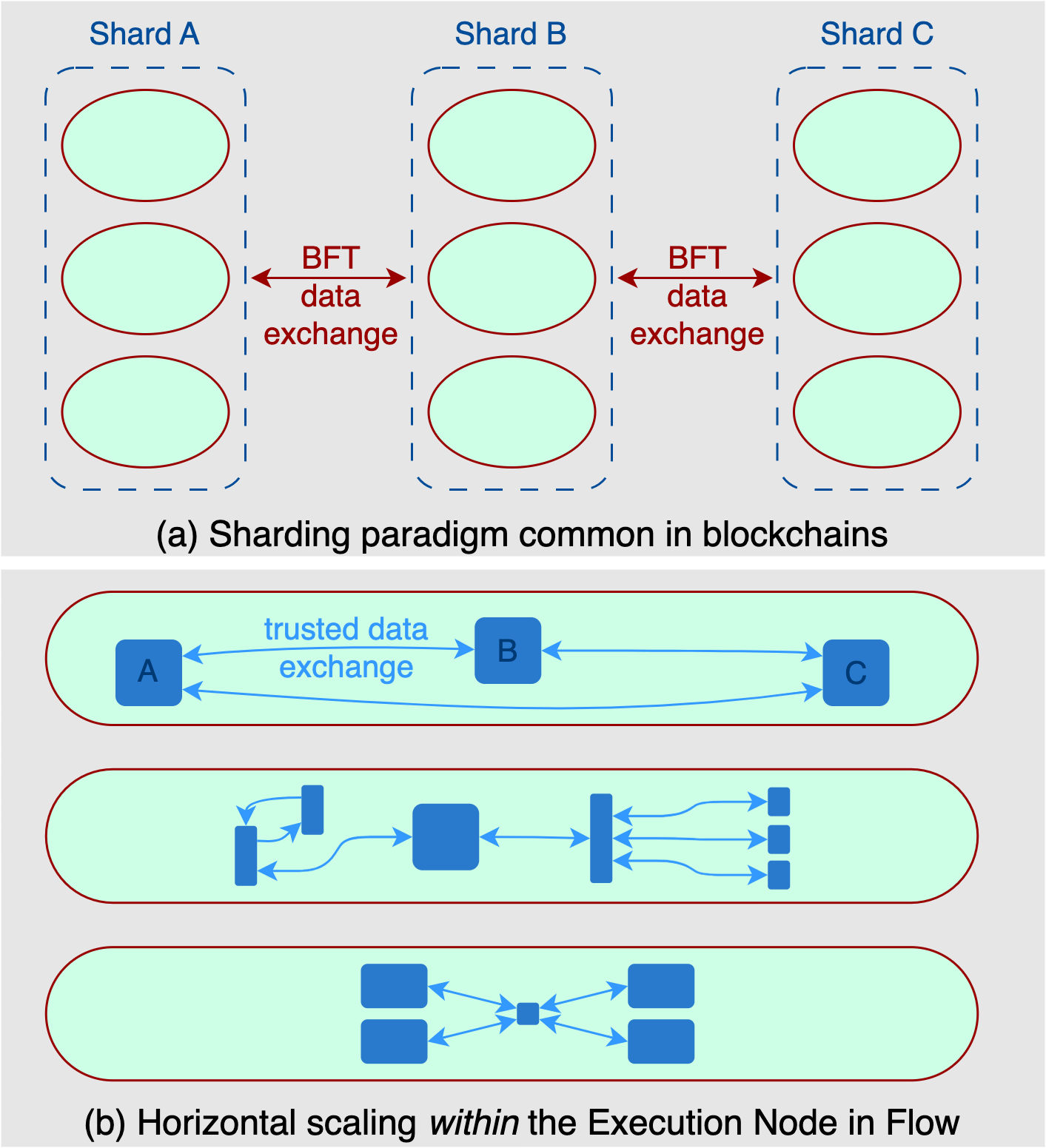
Figure (b) illustrates Flow’s horizontal scaling approach within the execution nodes (green ovals). Execution nodes have different heuristics for parallelizing the computation internally. For instance, the top execution node internally partitions the computation in a similar way to the shards from figure (a). However, as the data exchange is entirely internal to the node, there is no overhead. Moreover, different execution nodes implement different heuristics for horizontally scaling their computation. A diverse mix of scaling heuristics increases Flow’s resilience and alleviates load-specific bottlenecks of individual transaction parallelization heuristics.
In conclusion, there are massive efficiency and scalability benefits from concentrating transaction execution within a limited number of dedicated strong nodes. We highlight that Flow achieves this without compromising security or decentralization. This is because for a given starting state and a sequence of computational instructions, there is a single objectively-correct result. The verification step described in the following chapter guarantees that only correct results are accepted. Therefore, correctness of the system outputs is guaranteed despite low redundancy.
Distributed Verification
We have argued that for a highly-efficient, scalable compute platform, redundancy must be carefully controlled to keep resource consumption practical. However, with low redundancy, the majority (or all) execution nodes being compromised becomes a plausible scenario. To guarantee that only correct transaction execution results are accepted, we utilize redundancy. Essentially, we are asking a group of nodes, called ‘Verification Nodes’ or ‘Verifiers’ in short, to re-run the computation. If a larger fraction of Verifiers agree with the result, Flow will consider the result as correct and otherwise reject it as faulty.
Observant readers will notice that we are reducing redundancy of execution nodes to be more efficient but then adding redundancy at the verification step to prevent faulty results to slip though. Nevertheless, by substantially cutting execution redundancy we can dramatically reduce energy and hardware consumption. But we only need to add a little bit of redundancy, with limited hardware and energy cost, at the verification state. With such an architecture, the entire platform is still substantially more efficient than having high redundancy at the execution state.
To illustrate the underlying idea, consider a hypothetical scenario where evil Eve and byzantine Bob are contractors installing a fire alarm and trying to get away with a bad, incorrect job. The established process is that at the end Eve and Bob will have to show their work to a licensed inspector, who has to sign off. In simplified terms, we have a redundancy of 3, because Eve, Bob and the inspector are all spending time. Eve and Bob are trying to bribe the inspector to sign off on their faulty work. Depending on the inspector, some might be vulnerable, accept the bribe and become byzantine, while other inspectors withstand the compromising offer. Assume we have 1000 licensed inspectors working in the city, ⅓ of which are vulnerable to be compromised. For one inspector checking the work, Eve and Bob have a statistical change of 33.3% to get away with their faulty result. To make it more challenging, we can assign α = 5 inspectors and require Eve and Bob to present signatures from at least ⅔ of them. In this case, they have to get lucky enough so that there are 3 vulnerable inspectors, which reduces the chance of accepting faulty work to less than 5%. Assigning 50 inspectors already decreases the probability to less than 1 in a million!
The crucial insight is that we don’t need all 1000 inspectors to check the work. We can still build a safe and efficient protocol, by randomly assigning a limited number of inspectors from a large cohort.
Conceptually, we apply the same approach to reject faulty execution result in Flow:
- Each execution node publishes their own result, essentially pledging a large safety deposit (in blockchain terms ‘stake’) to its result being correct.
- Consensus nodes record the unverified results in blocks and randomly assign α Verification nodes to check the result. Through a sophisticated cryptographic process (DFinity-style random beacon [4]), the verifier assignment is unknowable at the time the Execution Node publishes its result.
- ⅔ of the assigned Verifiers need to approve the execution result. If the verifiers find a fault in the result, the execution node’s stake is taken (formally ‘slashed’) as penalty; equally so, if the execution node doesn’t reveal computation details to the verification nodes enabling them to check.
Figure 7 shows the probability of accepting a wrong execution result depending on the number of assigned verifiers α. With 10 execution nodes and assigning α=100 verifiers, we have a redundancy of 110, which is comparatively small. Other blockchain systems have execution redundancy of several hundreds, even approaching 2000 [25]. In comparison, Flow’s redundancy for transaction execution is about one order of magnitude less. We emphasize that Flow limits redundancy only for execution, which does not undermine decentralization and resilience, as execution nodes solely compute the transactions in the order determined by the decentralized, highly-resilient parts of the network.
The only possible attack vector for Execution Nodes is to cease operations completely. Nonetheless, a single honest execution node is sufficient to keep Flow running.
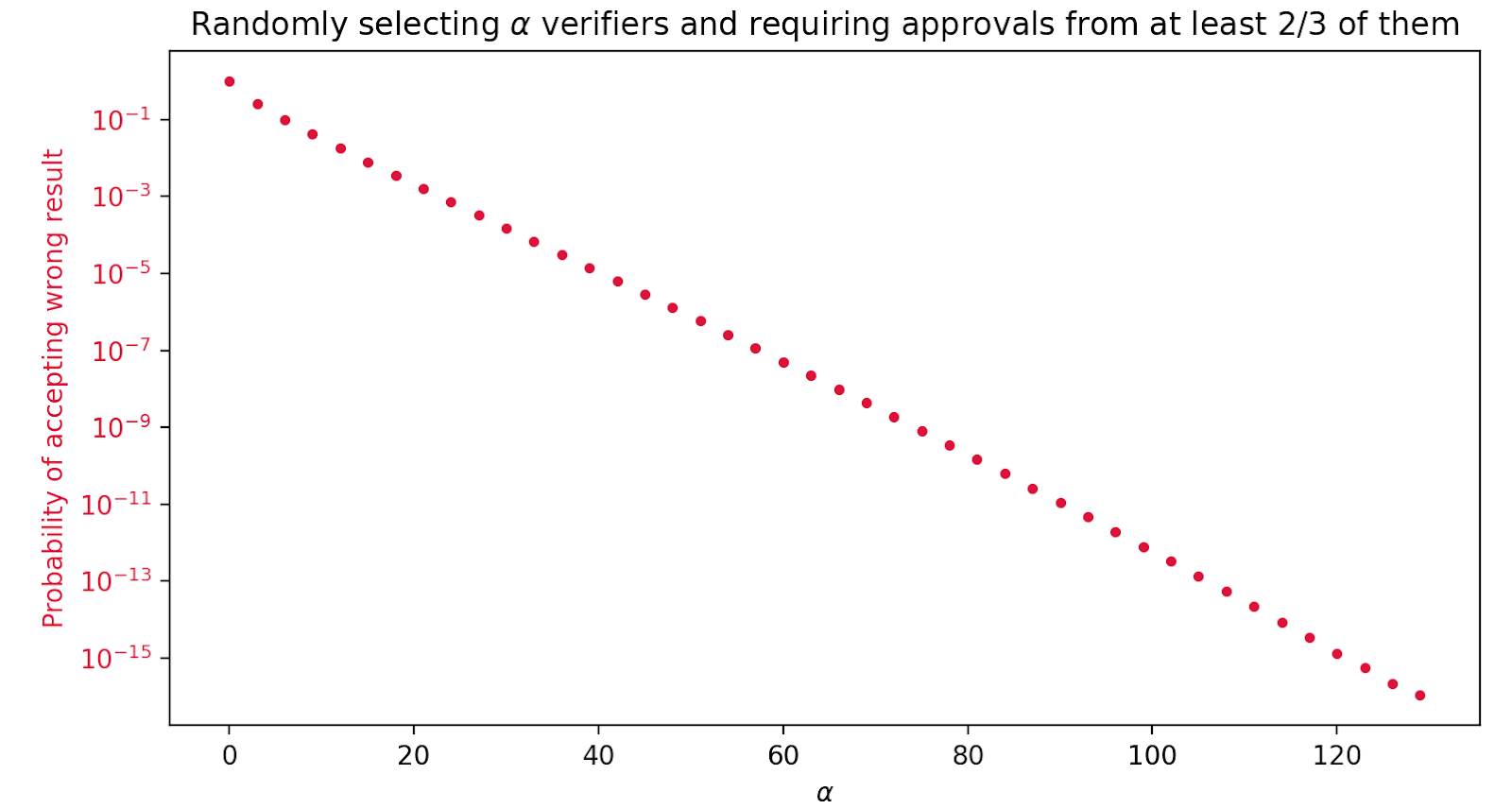
The description of Flow’s verification stage in this article is strongly condensed and simplified. For example, in the full protocol, we break the execution results into many small chunks. Each verification node only processes about 5 - 8% of all the chunks comprising a block. Thereby, the heavy work of checking a block is distributed and parallelized across the entire cohort of verification nodes.
For further details, we refer readers to our paper [26] on execution and verification. While the approach described in the paper still applies, some of the subtle technical details have been revised and refined. For the interested reader, we will publish an updated paper in the future.
Summary and Conclusions
Redundancy is a central parameter in a distributed compute platform, such as Flow. While high redundancy usually benefits resilience and decentralization, it also entails large hardware and energy consumption. For example, replicating 1 petabyte of state across hundreds to thousands of nodes is neither economically nor ecologically justifiable.
In our view, efficient scalability and support for highly-interacting, composable applications is quintessential for any distributed operating system aiming for mass-adoption and broad utility. Efficient scalability is an ongoing challenge and an area of active research and development. It is well-established that any general-purpose compute platform, from multi-core CPUs to cloud infrastructure, cannot be scaled without sacrificing efficiency or software composability, unless scalability is designed from the start into the platform. This holds for conventional compute platforms, which can tolerate crash failures. Extending the requirements to also tolerate byzantine failures makes scalability even harder. Therefore, I strongly disagree with the prevailing approach in the blockchain space to design systems with severely limited scalability and betting on future extensions (sharding, rollups, zero-knowledge proofs) to solve it.
For Flow, scalability is an intrinsic part of the architecture, considered at every step of development. On a technical level, we achieve unprecedented scalability by carefully controlling redundancy. Flow’s architecture separates processing steps across specialized node roles to individually optimize redundancy. Thereby, we achieve maximal efficiency without sacrificing decentralization or resilience. In particular transaction execution, the dominant driver of hardware and energy consumption, has exceptionally low redundancy in Flow. For example, in 2021 Flow consumed a total of 0.18 GWh in energy [7], which equals the power consumption of about 30 people [8]. Already today, Flow is among the most efficient BFT compute platforms in existence [9]. We stress that transaction throughput for Flow and other more-recent blockchain platforms is still orders of magnitude below their mature levels. As computational load increases, energy consumption will generally increase but we expect Flow to scale much more efficiently than other platforms due to its unique architecture.
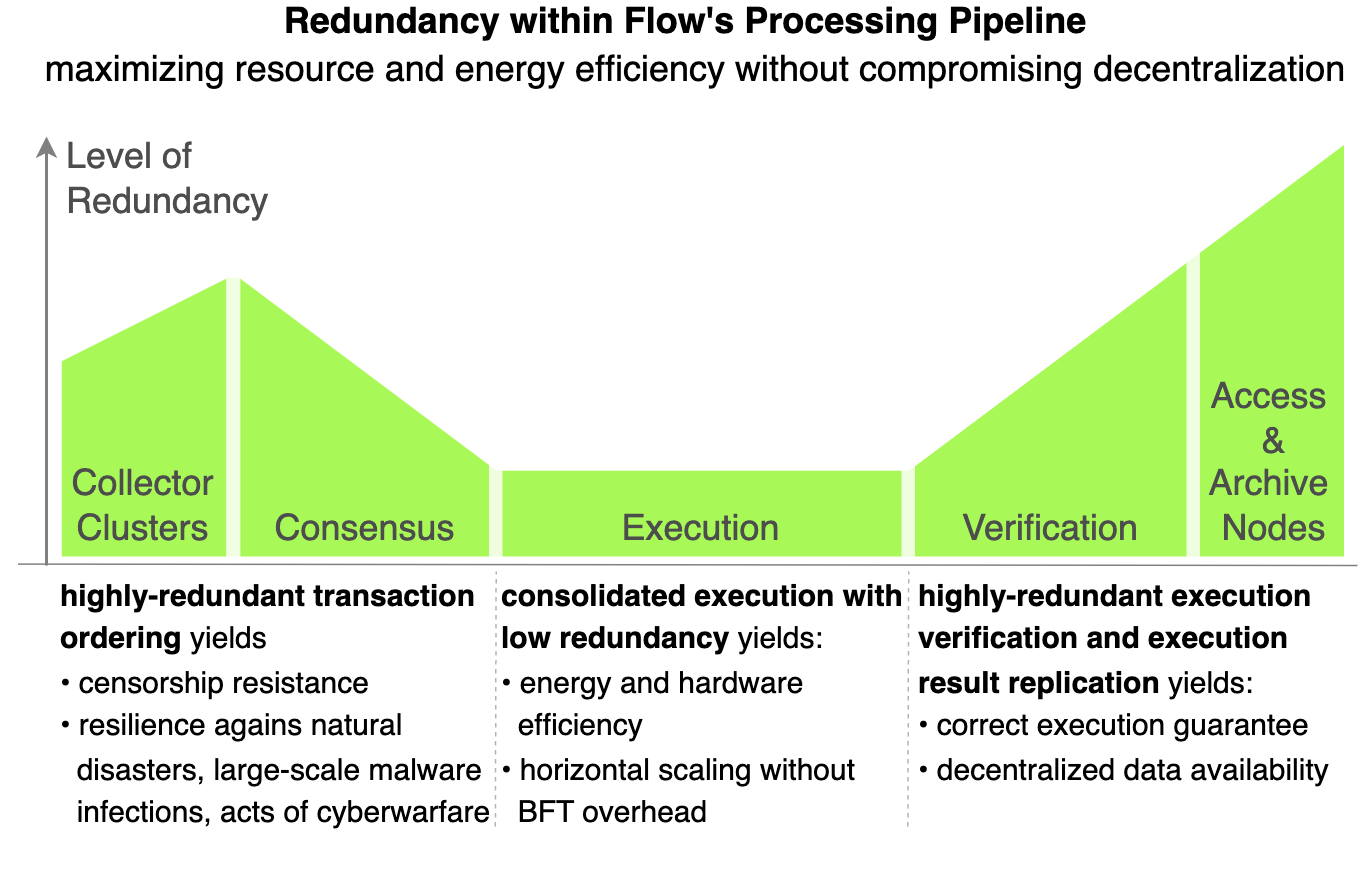
As a closing remark, we want to briefly discuss complexity. The Flow protocol might appear complex with its 5 different node roles compared to other popular blockchains. However, we emphasize that the Flow protocol already contains the entire complexity for scaling Flow to global adoption, while other projects delegate the scaling complexity into the future. For example, we concur that Ethereum 1.0 is substantially simpler than the Flow, but it does not scale. To tackle their scaling challenge, Ethereum 2.0 is by orders of magnitude more complex.
We conjecture that in a future comparison of Flow vs other protocols that have solved the scaling challenge (without compromising composability), Flow will be among the simplest and most intuitive protocols. This is because concentrating execution in dedicated, high-powered execution nodes is an innovation unique to Flow. Other protocols (including Ethereum 2.0, Near, etc) attempt to horizontally scale transaction execution across different nodes without trust relationships. Therefore, they must specify on a protocol level the detailed mechanics to horizontally scale transaction execution.
by Alexander Hentschel, PhD, Chief Protocol Architect of the Flow Blockchain, 2023
Stay up to date with the latest news on Flow.
Stay up to date with the latest news on Flow.